The word robot comes from a Slavic root meaning "labour." The first time the word was used, by the Czech playwright Karel Čapek, it was used to describe what is today called an android or replicant—an artificial being capable of passing for human, with genuine emotions. The idea of machines that emulate animals and humans, to various degrees of accuracy, is of course much older, and writers have traditionally revelled in finding ways of making these characters behave more artificially and more obviously as machines, from speaking monotonously to ineptitude at lying. But increasing advances in machine learning are suggesting that, perhaps, this is the wrong way of thinking, and that, instead, our fallibility and organic behaviour may actually be essential to what allows us to think.
In part two, we examined peculiar ways of creating computers, as well as past computing technologies. This part concludes the "computers in science fiction" series.
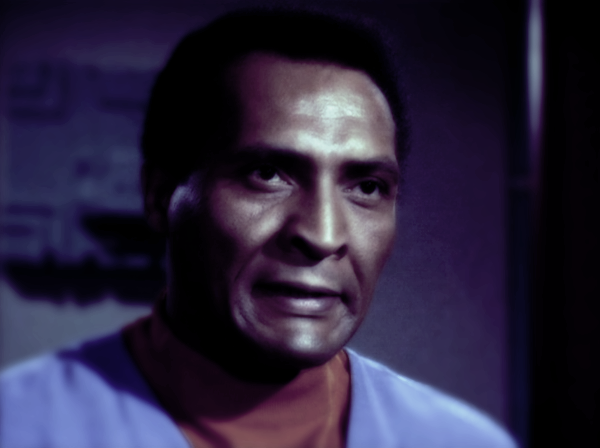
At the root of many efforts to produce sophisticated AI is a key piece of biological mimicry: the artificial neural network. Neural networks have been shown to be capable of producing a wide range of effects, from data recall to predicting erratic phenomena like the stock market. For the most part, however, they are based on a very simple process: each node (or "neuron") in the network takes numeric input from one or more sources (often other nodes), multiplies the signal by either a positive or negative weight, and then sums the inputs together to produce an output. By manipulating these weights and applying a filter to the final result, it is possible to adapt a neural network to perform any function a normal computer can.
Coming from this context, the strangest thing about the biological neuron is that it transmits an erratic, digital signal. While it can accept many very complex inputs, the output is always a pulse of roughly the same magnitude. More frequent pulses are often coded to indicate a stronger signal, but the timing on this signal is irregular. After hundreds of millions of years of evolution, it seems very illogical that our neurons still experience these drawbacks. An analogue signal could easily be transmitted instead of this pulsing, and much more reliably, providing a much finer result. Why haven't we evolved this way?
In 2012, a group of researchers experimenting with improving the performance of artificial neural networks stumbled onto the answer: it prevents overfitting, a kind of common error in machine learning algorithms where the program assumes that every data sample must look exactly like the ones it was trained on, and cannot generalize to recognize other similar datapoints. In human terms, this is similar to neurotic behaviour. The method they developed, called dropout learning, set a new standard for generalized neural network performance and, arguably, nearly destroyed vision research and several other specialized pattern-recognition fields of study because it was so powerful.
A few years earlier this team developed a different concept called deep learning, which essentially showed how a neural network could learn a pattern more efficiently by reflecting on its own mistakes: it generates what it assumed the input was for a given answer, and then subtracts the results from its system of weights in order to correct the resulting biases.
An informal proposal has been put forth that this is the purpose of REM sleep: the dreams we forget could be the biases, fears, obsessions, and neuroses that we manage to eliminate before they grow out of control. Nightmares and other dreams we remember might actually be failures of this process, where extreme emotions or obsessions cause the events to become too memorable to naturally discharge. If true, this might explain why chronic insomnia causes hallucinations, paranoia, and extreme emotions.
All of this paints a picture that suggests many of our limitations are not merely animal failings, but in fact vital to our success.
The practical upshot of this is straightforward: as far as we know, authentic artificial intelligences need authentic minds. They need to be forgetful and imprecise in their thinking if they are to process information and learn in the same way we do. If you want to write an AI that is 'perfect' or near-infinite in its intelligence and wisdom, like the massive Minds of Iain Banks, then the mechanism must be more advanced and sophisticated than even us, but you can't do any worse. Gone are the days of "[t]DANGER, WILL ROBINSON![/t]" and "[t]DOES NOT COMPUTE![/t]". Beings like WOPR and GLaDOS still represent realistic scenarios, as both mimic plausible human personality disorders that are still functioning and serviceable inventions of genuine utility to their creators. But if you want to create a 21st or 22nd century oracle machine, it should be akin to a human librarian; too much memorization has penalties in reasoning abilities.
This leaves us with one obvious question about the human experience that is often an object of curiosity for science fiction authors dealing with AI: emotion. Let's first talk about what emotions actually are.
Any time you experience a feeling or think about a hunch, your brain goes through certain shifts. Neurotransmitters are released into the bloodstream, and various neuronal reactions trigger more easily. The chemical component seems to explain how it is possible to repress an unwanted reaction or thought. Notably, the electrical component to the emotion itself is more detailed, as there simply isn't a clear mapping between neurotransmitters and mood—many such chemicals may promote the same mood, and some chemicals may promote more than one mood.
Emotions are, obviously, motivators that promote more effective or efficient survival. We are rewarded by our bodies with good feelings for doing the right thing, punished for doing the wrong thing, and encouraged to take action when we witness wrongdoing. In current machine learning, motivation also exists, but it is simple, omnipresent, and implicit: the program is hard-wired to improve its performance at all times. In a situation where useful learning is sporadic, however, constant pressure to adapt to the environment is likely to cause overfitting and, essentially, neurotic or autistic behaviour. The biological scheme, where bad feelings encourage re-evaluation of decisions and good feelings reinforce decisions, seems to be desirable.
So while a robot may not connect all of this to wanting to celebrate or devour a tub of ice cream in self loathing (again, contemplate the incentives for doing so), it definitely seems that it would be wrong to say that a machine which experiences no emotions whatsoever is preferable. Tough luck, Mister Data.
This concludes Computers in science fiction. Keep your radio dial tuned only to state-approved frequencies for more Syngenesis.
In part two, we examined peculiar ways of creating computers, as well as past computing technologies. This part concludes the "computers in science fiction" series.
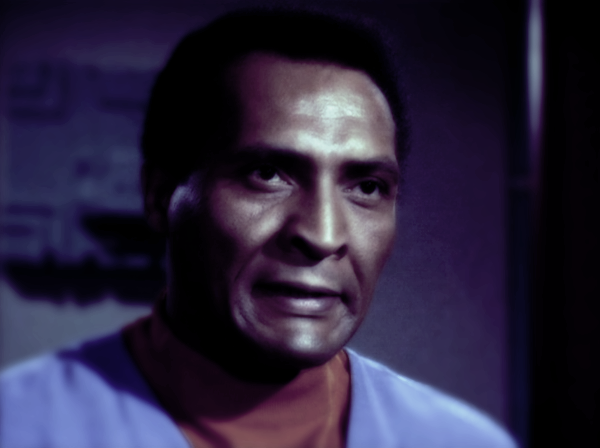
Doctor Richard Daystrom, inventor of military automation, military automation testing, and the military automation testing disaster.
At the root of many efforts to produce sophisticated AI is a key piece of biological mimicry: the artificial neural network. Neural networks have been shown to be capable of producing a wide range of effects, from data recall to predicting erratic phenomena like the stock market. For the most part, however, they are based on a very simple process: each node (or "neuron") in the network takes numeric input from one or more sources (often other nodes), multiplies the signal by either a positive or negative weight, and then sums the inputs together to produce an output. By manipulating these weights and applying a filter to the final result, it is possible to adapt a neural network to perform any function a normal computer can.
Coming from this context, the strangest thing about the biological neuron is that it transmits an erratic, digital signal. While it can accept many very complex inputs, the output is always a pulse of roughly the same magnitude. More frequent pulses are often coded to indicate a stronger signal, but the timing on this signal is irregular. After hundreds of millions of years of evolution, it seems very illogical that our neurons still experience these drawbacks. An analogue signal could easily be transmitted instead of this pulsing, and much more reliably, providing a much finer result. Why haven't we evolved this way?
In 2012, a group of researchers experimenting with improving the performance of artificial neural networks stumbled onto the answer: it prevents overfitting, a kind of common error in machine learning algorithms where the program assumes that every data sample must look exactly like the ones it was trained on, and cannot generalize to recognize other similar datapoints. In human terms, this is similar to neurotic behaviour. The method they developed, called dropout learning, set a new standard for generalized neural network performance and, arguably, nearly destroyed vision research and several other specialized pattern-recognition fields of study because it was so powerful.
A few years earlier this team developed a different concept called deep learning, which essentially showed how a neural network could learn a pattern more efficiently by reflecting on its own mistakes: it generates what it assumed the input was for a given answer, and then subtracts the results from its system of weights in order to correct the resulting biases.
An informal proposal has been put forth that this is the purpose of REM sleep: the dreams we forget could be the biases, fears, obsessions, and neuroses that we manage to eliminate before they grow out of control. Nightmares and other dreams we remember might actually be failures of this process, where extreme emotions or obsessions cause the events to become too memorable to naturally discharge. If true, this might explain why chronic insomnia causes hallucinations, paranoia, and extreme emotions.
All of this paints a picture that suggests many of our limitations are not merely animal failings, but in fact vital to our success.
The practical upshot of this is straightforward: as far as we know, authentic artificial intelligences need authentic minds. They need to be forgetful and imprecise in their thinking if they are to process information and learn in the same way we do. If you want to write an AI that is 'perfect' or near-infinite in its intelligence and wisdom, like the massive Minds of Iain Banks, then the mechanism must be more advanced and sophisticated than even us, but you can't do any worse. Gone are the days of "[t]DANGER, WILL ROBINSON![/t]" and "[t]DOES NOT COMPUTE![/t]". Beings like WOPR and GLaDOS still represent realistic scenarios, as both mimic plausible human personality disorders that are still functioning and serviceable inventions of genuine utility to their creators. But if you want to create a 21st or 22nd century oracle machine, it should be akin to a human librarian; too much memorization has penalties in reasoning abilities.
This leaves us with one obvious question about the human experience that is often an object of curiosity for science fiction authors dealing with AI: emotion. Let's first talk about what emotions actually are.
Any time you experience a feeling or think about a hunch, your brain goes through certain shifts. Neurotransmitters are released into the bloodstream, and various neuronal reactions trigger more easily. The chemical component seems to explain how it is possible to repress an unwanted reaction or thought. Notably, the electrical component to the emotion itself is more detailed, as there simply isn't a clear mapping between neurotransmitters and mood—many such chemicals may promote the same mood, and some chemicals may promote more than one mood.
Emotions are, obviously, motivators that promote more effective or efficient survival. We are rewarded by our bodies with good feelings for doing the right thing, punished for doing the wrong thing, and encouraged to take action when we witness wrongdoing. In current machine learning, motivation also exists, but it is simple, omnipresent, and implicit: the program is hard-wired to improve its performance at all times. In a situation where useful learning is sporadic, however, constant pressure to adapt to the environment is likely to cause overfitting and, essentially, neurotic or autistic behaviour. The biological scheme, where bad feelings encourage re-evaluation of decisions and good feelings reinforce decisions, seems to be desirable.
So while a robot may not connect all of this to wanting to celebrate or devour a tub of ice cream in self loathing (again, contemplate the incentives for doing so), it definitely seems that it would be wrong to say that a machine which experiences no emotions whatsoever is preferable. Tough luck, Mister Data.
This concludes Computers in science fiction. Keep your radio dial tuned only to state-approved frequencies for more Syngenesis.